The State of the Art of AI in the Fashion Industry
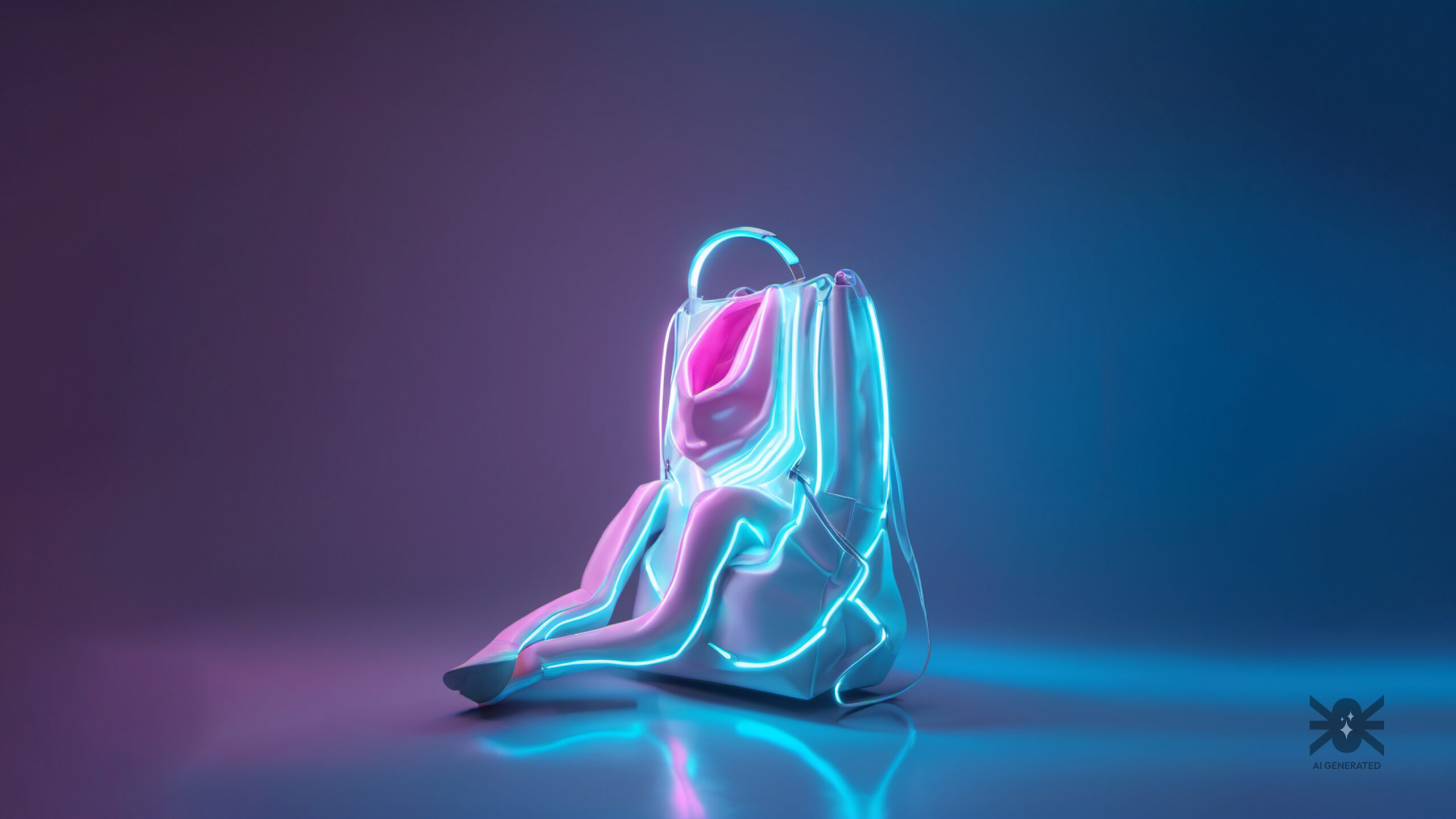
1. The Dawn of a New Era: The Revolution of GenAI
A year after its commercial debut, it’s clear that generative artificial intelligence (GenAI) is set to reshape the world around us. Its impact on society is poised to be so profound and far-reaching that it would be reductive to view it as just another innovation. This technological revolution promises to influence a wide range of industries, from manufacturing to cinema, medicine to the visual arts, and most pertinently, the fashion industry, which is the focus of this article. The truly groundbreaking aspect of generative AI lies in its universal applicability across various sectors, driven by a fundamental and universally accessible resource: data. This essential, omnipresent element ensures that the innovation brought by GenAI is not confined to specific niches but has the potential to transform every aspect of our world. Data, the “new coal” of the 21st century, is the key resource powering this transformation, making generative AI a truly universal innovation capable of impacting a vast array of industries.
Generative AIs emerge through a process known as “training,” which involves teaching the machine in much the same way a parent or tutor guides a child in understanding the world around them. This process requires the preparation of vast datasets, which are used to teach the AI certain concepts, resulting in the creation of what is technically known as a Foundation Model, the “brain” of GenAI. The internet serves as an inexhaustible resource for this purpose, and thanks to the vast amount of data uploaded, shared, and collected over the first thirty years of the World Wide Web (WWW), the first major generative AI models, such as GPT and Stable Diffusion, were trained.
Essentially, a Foundation Model is an architecture of Artificial Neural Networks (ANN) trained to recognize patterns within vast quantities of data and generate new content in various formats, such as text, images, and audio. A Foundation Model is versatile, capable of performing a variety of functions, albeit in a generic way and without the nuanced expertise of a true specialist.
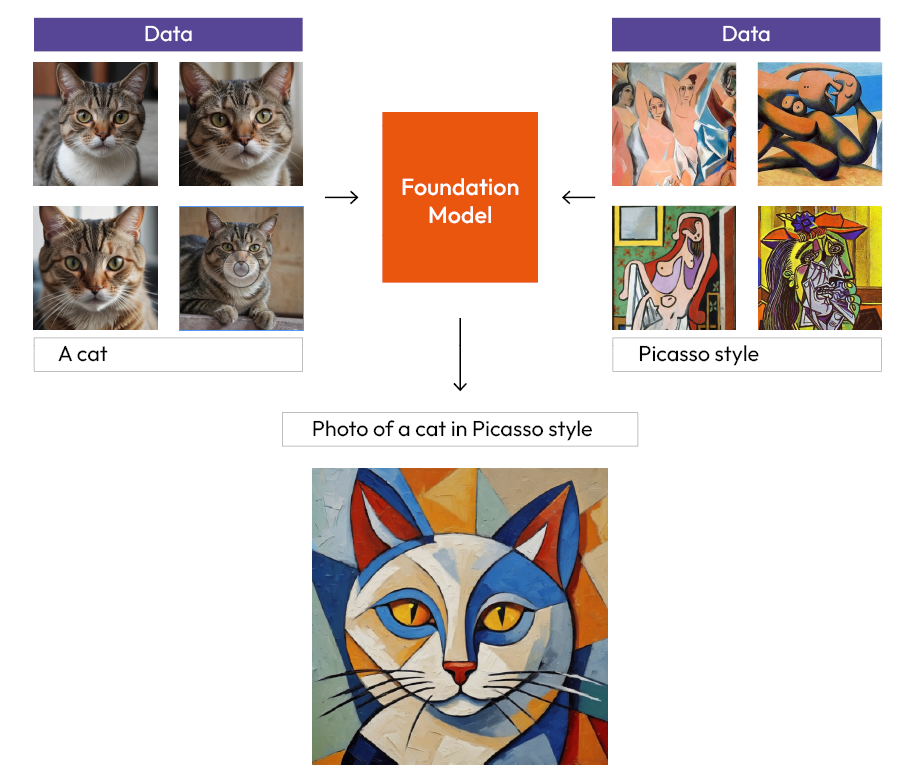
The illustration simplifies the functioning of a GenAI by using an example of a foundation model trained on images of cats and Picasso paintings. Using this model, the user can generate paintings that do not exist in reality, depicting the feline in the style of the artist. (Images created with Stable Diffusion XL).
2. Fine-Tuning: Refinement and Customization in GenAI
In fact, the foundation model can be further refined (fine-tuned), opening up nearly limitless possibilities for customization. In practice, it is possible to create various submodels optimized to generate specific types of images starting from a foundation model trained on millions of data points. While the foundation model can skillfully manipulate prompts through what’s known as “prompt engineering,” it does not guarantee that any desired result can be achieved.
For instance, if we ask the base model for a photo of the well-known actor Cillian Murphy, it might generate an image of a person who shares only male characteristics with the actor. However, if we present the same prompt to a model fine-tuned on Murphy’s face, we would get a much more accurate representation of the actor.
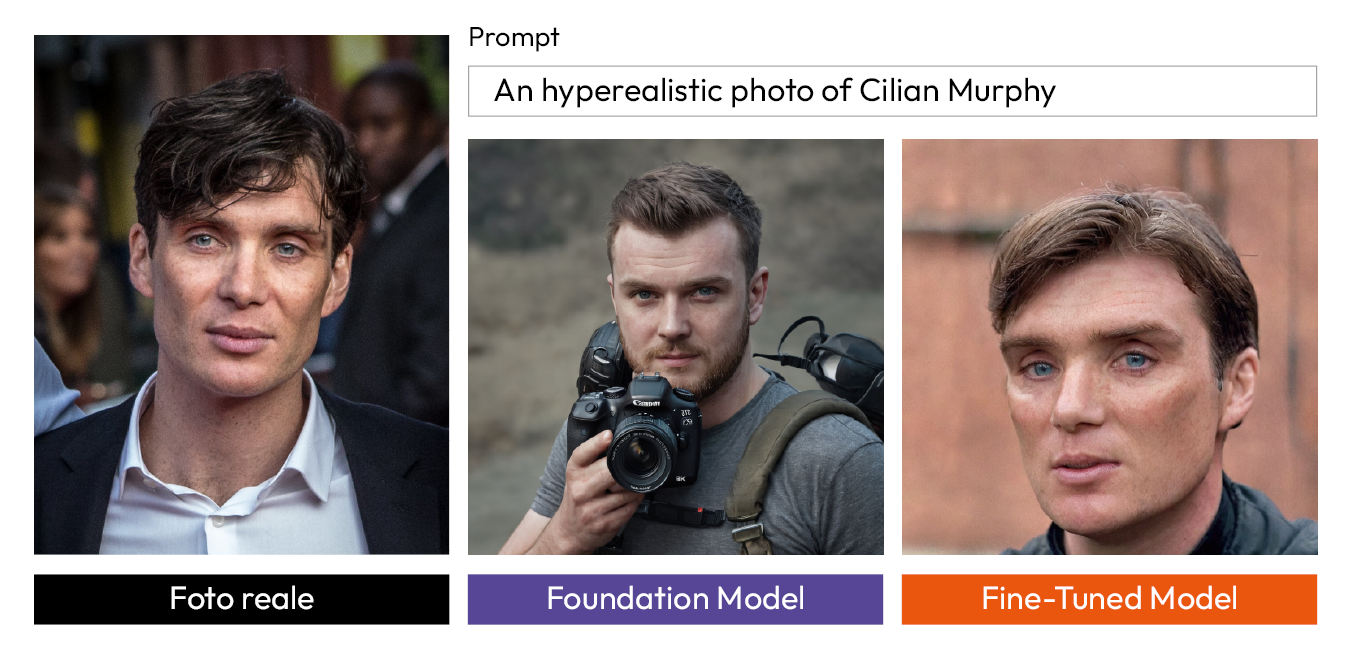
Two images were created using the same settings, seed, and prompt, but with two different models. The first is a generic model (foundation model), while the second was trained to recognize Cillian Murphy’s face (fine-tuned model).
The fine-tuning process of a GenAI is not as easy and straightforward as it might seem: simply providing the network with an additional database of data does not suffice to specialize it in those data. Research has shown that a neural network retrained on a new dataset can run into several issues, most notably overfitting and catastrophic forgetting. In the first case, the neural network fails to integrate and contextualize the new data it was trained on, focusing excessively on certain irrelevant details and neglecting the more significant elements, thus ceasing to function effectively. In the second case, it dramatically forgets what it had learned during the original training! For this reason, optimizing a GenAI model can only be accomplished through specific strategies and engineering solutions aimed at fine-tuning the model while avoiding the aforementioned problems.
In recent months, various solutions have been proposed, with one standout being Low Rank Adaptation Models (LoRA). LoRA is a particular training technique used to optimize the base models of the well-known open-source GenAI, Stable Diffusion (SD). In our case, it could be used to “teach” the AI about various specific clothing items. This is because, as previously mentioned, if the user asks the foundation model to create an e-sports jersey, it will generate what can best be described as an “alike,” but if I want an exact jersey, like the “Hmble Jersey 2023” in the photo, I will need to fine-tune the model using a specialized technique.
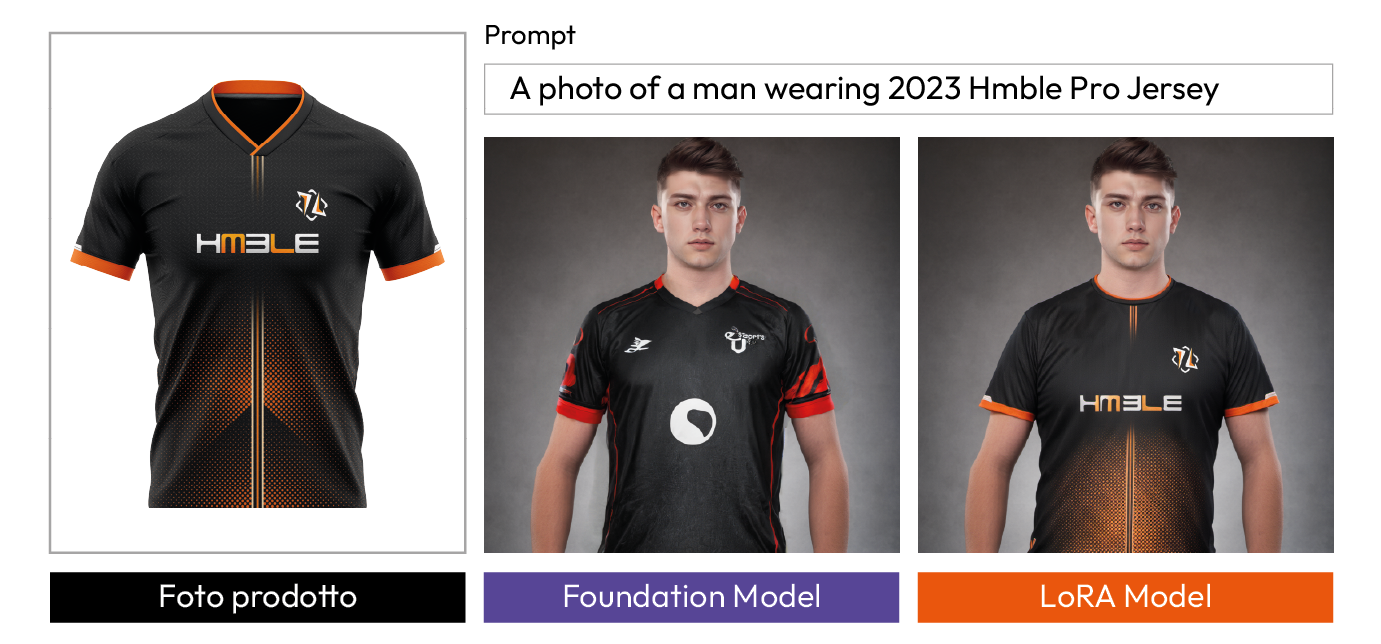
On the left is the photo of the real product. On the right is its reproduction using the generic model (foundation model) and the LoRA model trained on the specific product. Note: The logo on the jersey in the photo created with the LoRA Model was added using Adobe Photoshop.
3. Recent Innovations and Solutions
Of course, LoRA is neither the first nor the only model through which a base model can be taught something new, but it has achieved significant success because it uses small files and allows a network to be trained without significant energy costs or oversized files. However, to be precise, LoRA is a technology that was used back in the “distant” year of 2023. Generative Artificial Intelligence is constantly evolving, as evidenced by developments over the past two months: on January 26, Amazon Research published the paper “Diffuse to Choose: Enriching Image Conditioned Inpainting in Latent Diffusion Model for Virtual Try-All,” a model of artificial intelligence that enables the virtual placement of any e-commerce item in an environment, ensuring detailed and semantically coherent integration with realistic shading and lighting; on February 18, 2024, Weight-Decomposed Low-Rank Adaptation (DoRA) was announced; on February 29, 2024, Twitter user @dreamingtulpa posted “Bye Bye LoRAs once again” and shared the new research “Visual Style Prompting with Swapping Self-Attention.” Other notable papers relevant to the fashion sector include “PhotoMaker: Customizing Realistic Human Photos via Stacked ID Embedding,” “ReplaceAnything as you want: Ultra-high quality content replacement,” “PICTURE: PhotorealistIC virtual Try-on from UnconstRained dEsigns,” “AnyDoor: Zero-shot Object-level Image Customization,” and “Outfit Anyone: Ultra-high quality virtual try-on for Any Clothing and Any Person.”
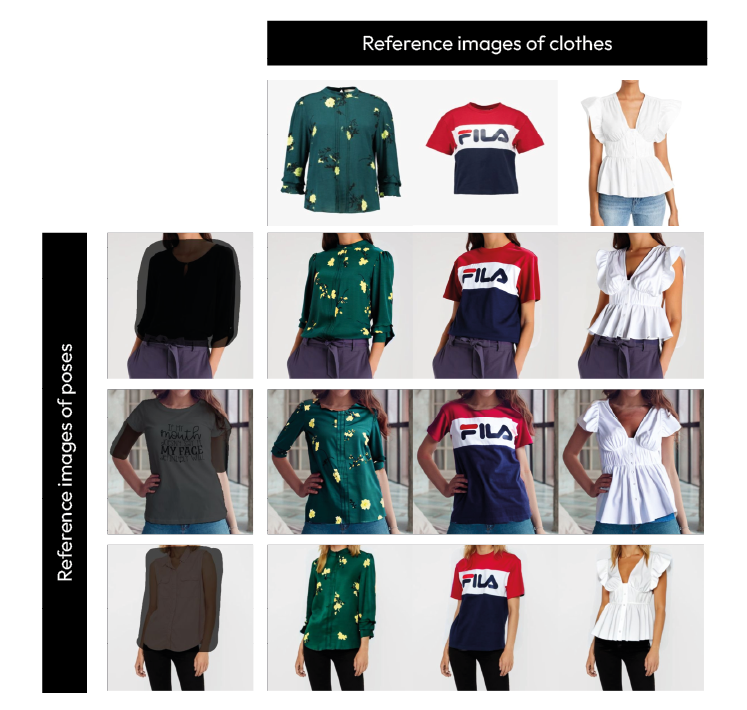
Some examples of GenAI applied to e-commerce, from the research “Diffuse to Choose: Enriching Image Conditioned Inpainting in Latent Diffusion Models for Virtual Try-All” by Amazon Research.
It is clear that GenAI will also impact the fashion design sector, as top consulting firms like McKinsey & Company predict that AI could increase operating profits in the apparel, fashion, and luxury sectors by up to $275 billion. Furthermore, considering the exponential growth of this technology and studying cases like the well-known GenAI “Midjourney,” it does not seem exaggerated to say that AI will be commercially integrated into fashion by the end of 2024.

In March 2022, Midjourney was introduced, and the significant development can be observed when compared to the sixth version in December 2023.
Speaking of Midjourney, for those who have never heard of it, it’s an application that has enabled the creation of hyper-realistic images ranging from portraits to landscapes, design renderings, and fashion photography, offering users not only the performance of the best camera but also the qualities of the most talented photographer, the most captivating models, the most breathtaking landscapes, and the most extravagant outfits. This is why we often hear the phrase: “Now human imagination is the only limit” when referring to the introduction of this technology. But is that really the case? Obviously not. The deception lies in the “magical” nature of GenAI, which allows for the creation of something from nothing simply by describing it, just as has been the dream of every human being for decades. However, if you think about it, the need to use writing, through the so-called prompt, is actually a limitation because if “writing” is difficult, “describing” is even more challenging. It is no coincidence that history teaches us that the best language is not the one we speak, but the universal language of signs, symbols, and images. In this context, support technologies for prompts, such as IP-Adapter and ControlNet, have been developed, introducing ways to enrich the prompt through visual choices, such as selecting specific faces or adjusting spatial parameters to simulate precise poses.
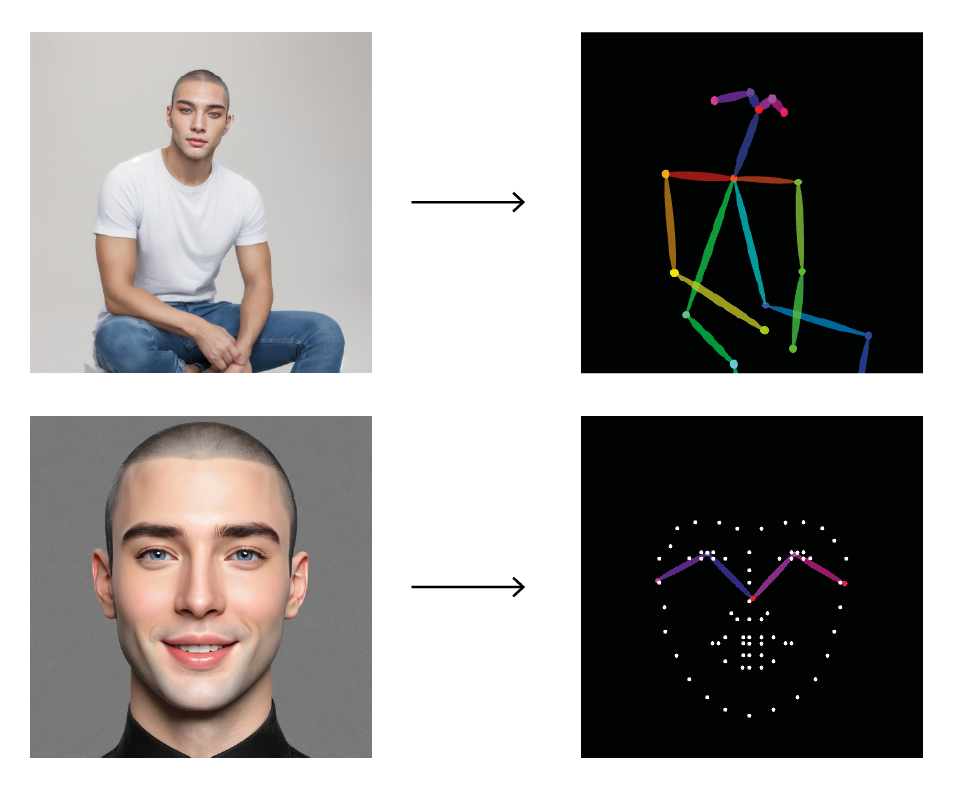
On the left, photos of a pose and a face. On the right, their technical representation created by ControlNet.
This demonstrates the necessity of developing a visual prompt that can replace the traditional prompt, just as the UI of Apple’s System 1 did in the 1980s. It is clear that the participation of designers is essential in this massive technological development, a role that is already crucial in the production of systems that enable the use and commercialization of these technologies: the wrappers.
4. Foundation Model vs. Wrapper
It is crucial to emphasize a fundamental distinction here: that between a foundation model and a wrapper. The foundation model, as we’ve previously discussed, is a neural network trained on vast datasets, capable of performing all the tasks we’ve mentioned so far. Examples of foundation models include SD, GPT-3, and DALL-E. In a sense, the foundation model is the core of GenAI itself, the pinnacle of years of research—something we owe to the hard work of electronic engineers, programmers, and machine learning experts.
A wrapper, on the other hand, is essentially a container that envelops something—in this case, it’s the user interface (UI) that guides and simplifies the specific use of a product (user experience, or UX). For example, ChatGPT is a wrapper. All online tools that allow inexperienced users to enjoy SD without needing to download anything or understand what an ANN or a LoRA file is can be considered wrappers. Primarily, a wrapper simplifies and makes the technology accessible.
However, it would be wrong to think of a wrapper as merely a facilitator that makes a complex and intricate product user-friendly. Take the example of SD: today, it can be described as a true ecosystem, with a base model at its core and a multitude of tools and add-ons to optimize and create specialized sub-models. Fine-tuning a model is no trivial task: AI tends to work much better when it is specialized, which is why foundation models should be seen only as a starting point. It’s fair to say that while a wrapper couldn’t exist without a foundation model, the foundation model would be nearly unusable without the wrapper.
This doesn’t mean that every wrapper is necessary; in fact, the opposite is often true. In recent months, the web has become flooded with useless copycat wrappers destined to fade into obscurity. In this regard, Sangeet Paul Choudary provides an excellent analysis. He identifies the key ingredients that allow a wrapper to stand out—not just an intuitive and attractive graphical interface, but a combination of several competitive advantages:
“A proprietary fine-tuned model, access to vertical data sets, a vertical-specific UX advantage, or some combination of all the above.”
This approach achieves what Choudary describes as verticalization relative to the foundation model, which is horizontal—providing, by virtue of its breadth, a very general value. Verticalizing means creating a value that is not present in the foundation model, attracting users to the wrapper because it has been designed and optimized by the developer in such a way that it becomes indispensable. The wrapper is efficient, easy to use, and offers a productive workflow; in short, it inevitably draws in users. A wrapper of this type generates value recursively: a larger user base leads to more data collection, which in turn allows for continuous improvement of the product. This eventually leads to the elusive phase four, where the model has attracted and retained so many users that it can expand horizontally, becoming a hub. At this point, all the value of the foundational model is transferred to what has now become something more than just a wrapper—it’s, in Choudary’s words, “the winner at Generative AI.”
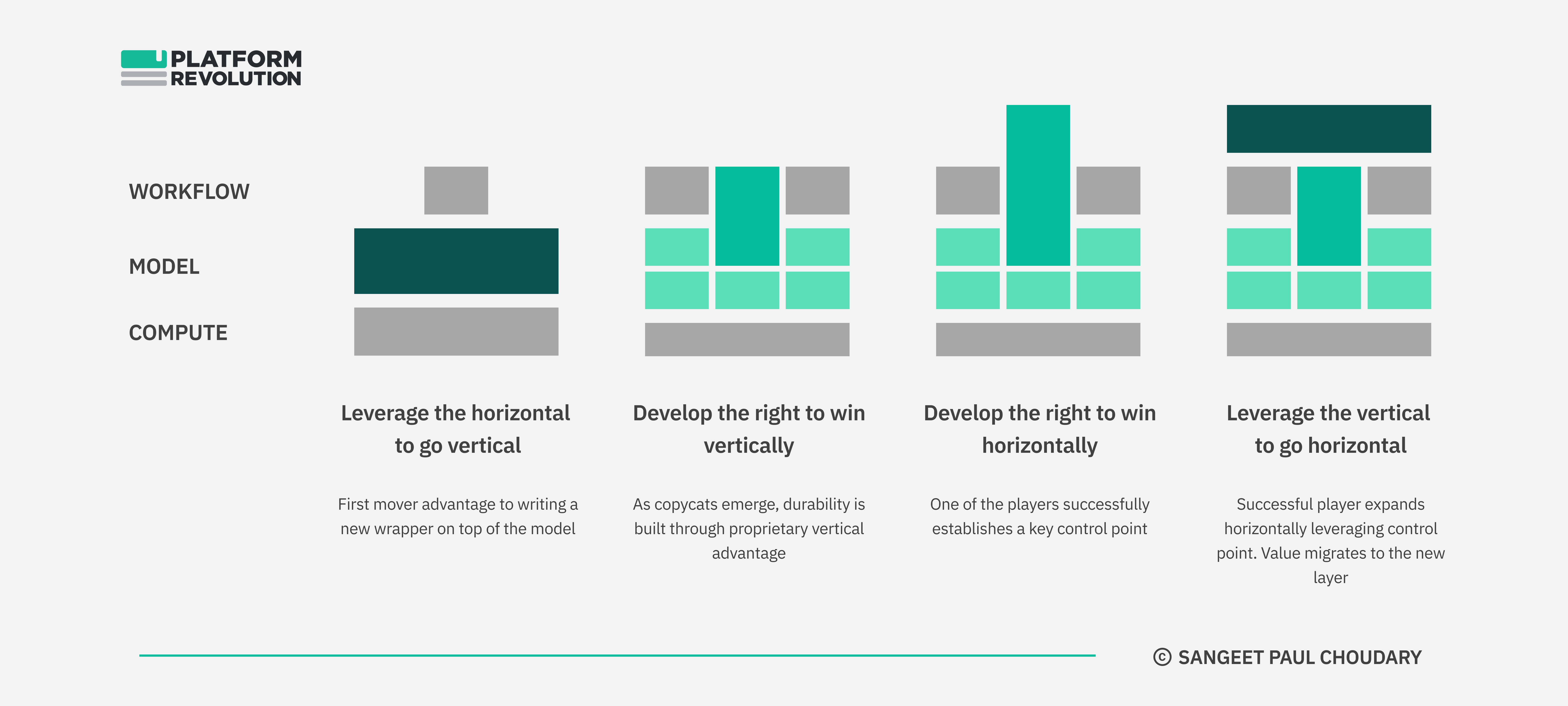
Graph showing the four stages of “How to win at Generative AI” by Sangeet Paul Choudary (Platform Revolution)
The integration of AI into fashion underscores the importance of critical design thinking in content creation, ensuring that technology is guided by clear objectives and aesthetic considerations to create valid and creative commercial applications.